Google DeepMind has announced an impressive suite of new products and prototypes that could help it regain its lead in the race to turn generative AI into a mass solution.
The big highlight is Gemini 2.0 — the latest version of Google DeepMind’s family of multimodal language models, now redesigned with a focus on the ability to control agents — and a new version of Project Astra, the experimental “all in one” app that the company presented at the Google I/O event in May.
MIT Technology Review had the opportunity to test Astra in a closed demo. The experience was impressive, but there’s a significant difference between a polished promotional video and a live demo.
Astra uses the agent framework built into Gemini 2.0 to answer questions and perform tasks through text, speech, image and video, connecting to Google apps like Search, Maps and Lens whenever necessary. “We are combining some of the most powerful information retrieval systems of our time,” says Bibo Xu, product manager at Astra.
Gemini 2.0 and Astra are accompanied by Mariner, a new agent that can browse the web for you; Jules, a programming assistant powered by Gemini; and Gemini for Games, an experimental assistant that can offer tips while you play video games.
(And it’s worth remembering that Google DeepMind also announced Veo, a video generation model; Imagen 3, the new version of its image generation model; and Willow, a new type of chip for quantum computers. Phew! Meanwhile, CEO Demis Hassabis was in Sweden yesterday accepting his Nobel Prize.)
Google DeepMind claims that Gemini 2.0 is twice as fast as the previous version, Gemini 1.5, and outperforms its predecessor on several standard benchmarks, including MMLU-Pro, an extensive set of multiple-choice questions used to test skills of great language models in diverse areas, from mathematics and physics to health, psychology and philosophy.
However, the differences between leading models such as Gemini 2.0 and those developed by competing laboratories such as OpenAI and Anthropic are now very small. Currently, advances in language models are not so much about the quality itself, but about what is possible to do with them.
And that’s where agents come in.
Hands-on with Project Astra
I was led through an inconspicuous door on an upper floor of a building in London’s King’s Cross district to a room that gave off strong “secret project” vibes. The word “ASTRA” was printed in giant letters on one of the walls. Xu’s dog, Charlie — the project’s unofficial mascot — walked among the tables where researchers and engineers were working on building the product on which Google is betting its future.
“The way I explain it to my mom is that we are creating an AI with eyes, ears and a voice. She can be anywhere with you and help with anything you are doing,” says Greg Wayne, one of the Astra team leaders. “We’re not there yet, but that’s the vision.”
The official term for what Xu, Wayne and their colleagues are developing is “universal assistant.” They’re still defining exactly what that means.
At the far end of the Astra room, there were two scenarios used for demonstrations: a drinks bar and a simulated art gallery. Xu took me to the bar first. “A long time ago, we hired a cocktail expert to teach us how to make drinks,” said Praveen Srinivasan, another project leader. “We record these conversations and use that to train our initial model.”
Xu opened a cookbook to a page with a recipe for chicken curry, pointed his cell phone at her and activated Astra.
“Ni hao, Bibo!” said a female voice.
“Oh! Why are you talking to me in Mandarin?” Xu asked on his cell phone. “Can you speak to me in English, please?”
“My apologies, Bibo. I was following a previous instruction to speak in Mandarin. I will now speak in English as requested.”
Astra remembers past conversations, Xu explained. It also keeps the last 10 minutes of video in memory. (In the promotional video released by Google in May, there is a striking moment where Astra tells the person where they had left their glasses, having seen them on a table seconds before. But I didn’t see anything like that during the live demo. )
Back to the cookbook. Moving his phone’s camera over the page for a few seconds, Xu asked Astra to read the recipe and tell him which spices were on the list.
“I remember the recipe mentions a teaspoon of black peppercorns, a teaspoon of chili powder and a cinnamon stick,” he replied.
“I think you are forgetting some things,” Xu said. “Take another look.”
“You are correct — I apologize. I also see turmeric powder and curry leaves among the ingredients.”
Watching this technology in action, two things become immediately clear. First, it still has flaws and needs to be fixed. Second, these flaws can be easily fixed with a few words. Just stop the voice, repeat the instructions and move on. It feels more like guiding a child than dealing with broken software.
Next, Xu pointed his cell phone at a row of wine bottles and asked Astra to choose one that would go well with the chicken curry. He suggested a rioja and explained why. Xu asked how much the bottle cost. Astra responded that it would need to use Search to check online prices. A few seconds later, he returned with the answer.
We moved to the art gallery, where Xu showed Astra a series of canvases with famous paintings: the Mona Lisa, Munch’s Scream, a Vermeer, a Seurat, among others.
“Ni hao, Bibo!” the voice said again.
“You’re speaking to me in Mandarin again,” Xu said. “Talk to me in English, please.”
“My apologies, it seems I misunderstood. Yes, I will respond in English.” (I should have known better, but I swear I felt a slight ironic tone.)
It was my turn. Xu handed me her cell phone.
I tried to put Astra in a difficult situation, but he wouldn’t budge. I asked which famous art gallery we were in, but he refused to venture a guess. I asked why he had identified the paintings as replicas, and he began to apologize for the mistake (Astra apologizes a lot). I was forced to interrupt: “No, no—you’re right, it’s not a mistake. You are correct in identifying canvas paintings as fake.” I couldn’t help but feel a little bad: I had messed up an app that exists solely to please.
When it works well, the Astra is fascinating. The experience of starting a conversation with your phone about whatever you’re pointing to feels new and fluid. At a press conference held yesterday, Google DeepMind showed a video showing other uses of Astra: reading an email on your phone’s screen to find an access code (and remembering it later), pointing your phone at a bus moving and ask where he is going, or ask questions about a public artwork as you pass by it. This could become generative AI’s big killer app.
However, there is still a long way to go before most people have access to technology like this. There is no mention of a release date. Google DeepMind also shared videos of Astra working on a pair of smart glasses, but that technology is even further down the company’s priority list.
Mixing the pieces
For now, researchers outside of Google DeepMind are closely watching Astra’s progress. “The way things are coming together is impressive,” says Maria Liakata, an expert on large language models at Queen Mary University of London and the Alan Turing Institute. “It is difficult to reason with language alone, but here it is necessary to integrate images and other elements. This is not trivial.”
Liakata was also impressed by Astra’s ability to remember things he saw or heard. She works with what she calls long-term context, developing models to track information they have previously come into contact with. “This is exciting,” says Liakata. “Even being able to do this in a single modality is already impressive.”
However, she admits that much of her analysis involves assumptions. “Multimodal reasoning is extremely advanced,” she says. “But it’s very difficult to know exactly where they are, because they haven’t said much about the technology itself.”
For Bodhisattwa Majumder, a researcher who works on multimodal models and agents at the Allen Institute for AI, this is a central concern. “We absolutely don’t know how Google is doing this,” he says.
Majumder points out that if Google were a little more transparent about what it’s building, it would help consumers understand the limitations of the technology they may soon get their hands on. “People need to know how these systems work,” he says. “It is important that the user can see what the system has learned about them, correct errors or remove information they want to keep private.”
Liakata also worries about the privacy implications, highlighting that people could be monitored without their consent. “There are things that excite me and things that worry me,” she says. “There’s something unsettling about your phone becoming your eyes.”
“The impact these products will have on society is so great that this should be taken more seriously,” she continues. “But this has become a race between companies. This is problematic, especially since we have no agreement on how to evaluate this technology.”
Google DeepMind says it carefully analyzes privacy, security and safety issues in all of its new products. Your technology will be tested by teams of trusted users for months before being released to the public. “Obviously, we need to think about misuse. We need to think about what happens when something goes wrong,” says Dawn Bloxwich, the company’s director of responsible development and innovation. “There is enormous potential. The productivity gains are enormous. But there are also risks.”
No testing team can predict all the ways people will use or abuse a new technology. So what’s the plan for when the inevitable happens? Companies need to design products that can be decommissioned or recalled quickly if necessary, says Bloxwich: “If we need to make quick changes or take something out of circulation, we can do that.”
( fonte: MIT Technology Review)
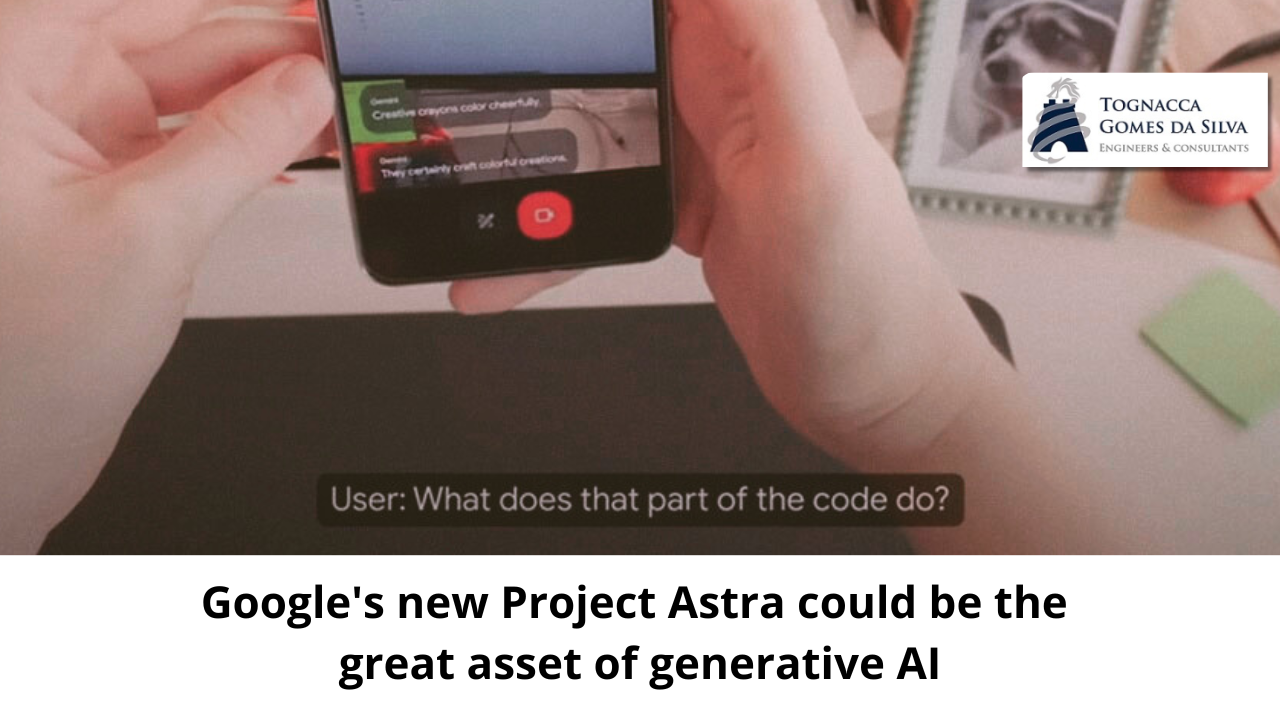