Meta has unveiled an AI model capable of translating speech from up to 101 languages, marking a significant step forward towards real-time simultaneous interpretation, in which words are translated as they are spoken. Typically, speech translation models follow a multi-step process: first, they convert speech to text; then, they translate the text into another language; Finally, they transform this translated text into speech in the target language. This method is susceptible to errors and inefficiencies at each step. However, Meta’s new model, called SeamlessM4T, allows for more direct translation between speech in different languages, as described in an article published today in Nature.
Seamless delivers 23% more accuracy in text translations compared to leading models. Although Google’s AudioPaLM supports more languages (113 versus 101 for Seamless), it only translates into English. SeamlessM4T translates into 36 other languages.
The model uses a process called parallel data mining, which identifies instances in which the sound of videos or audio matches subtitles in other languages, collected from the web. This allowed the model to associate sounds in one language with equivalent texts in another, substantially expanding its set of translation examples.
“The breadth of functions that Meta is developing is impressive, such as text to speech, speech to text and automatic speech recognition,” comments Chetan Jaiswal, professor of computer science at Quinnipiac University, who was not involved in the study. “The number of languages supported is a remarkable achievement.”
Despite innovations, the study states, human experts still play an essential role in the translation process, especially in dealing with cultural contexts and ensuring the accuracy of meaning between languages. Lynne Bowker, a researcher in the field at Université Laval, observes: “Languages reflect cultures, and cultures have their own forms of knowledge.”
Applications like medicine or law require machine translations to be rigorously reviewed by humans, he says. Otherwise, misunderstandings may occur. For example, in January 2021, Google Translate was used to translate public health information about the Covid-19 vaccine from the Virginia Department of Health. The tool interpreted “not mandatory” in English as “not necessary” in Spanish, completely changing the meaning of the message.
AI models have many more examples for training in some languages than others. This means that current speech-to-speech translation models can translate, for example, Greek into English, where there are many examples available, but cannot translate from Swahili into Greek. The team behind Seamless sought to solve this problem by pre-training the model with millions of hours of spoken audio in different languages. This allowed him to recognize general patterns in the language, making it easier to process less spoken languages, as the model had a prior basis of what a spoken language should sound like.
The system is open source, and the researchers hope this will encourage other developers to expand the model’s current capabilities. However, there is skepticism about its usefulness compared to available alternatives. “Google’s translation model isn’t as open as Seamless, but it’s much more responsive and faster, and it doesn’t cost anything for academics,” says Jaiswal.
The most exciting aspect of Meta’s system is that it points to the possibility of instantaneous interpretation across languages in the near future—like the Babel Fish in Douglas Adams’ cult novel The Hitchhiker’s Guide to the Galaxy. SeamlessM4T is faster than existing models, but it’s still not instantaneous. That said, Meta claims to have a newer version of Seamless that is as fast as human interpreters.
“While delayed translation is useful and has value, I believe simultaneous translation will be even more advantageous,” says Kenny Zhu, director of the Arlington Computational Linguistics Lab at the University of Texas at Arlington, who is not involved in the new research.
( fonte: MIT Technology Review)
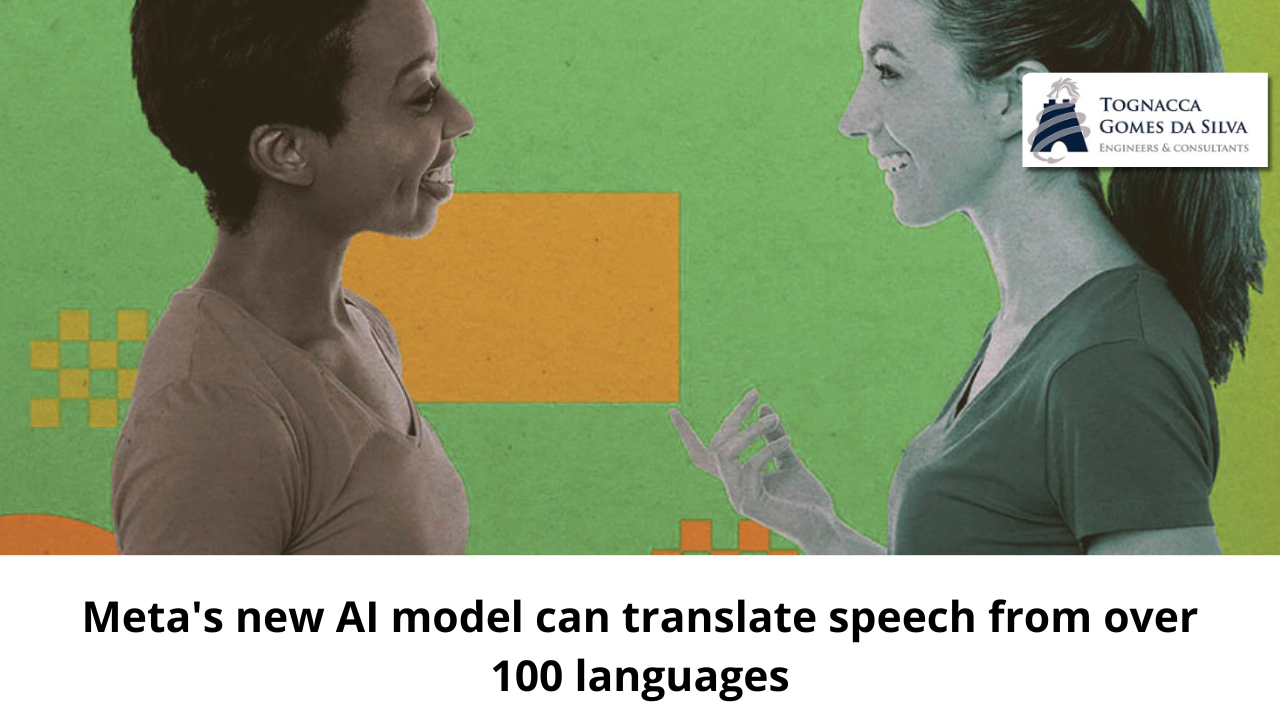