Google launched the first phase of its next-generation AI model, Gemini, on December 6. The tool reflects years of efforts within the company, overseen and led by its CEO, Sundar Pichai.
Pichai, who previously oversaw Chrome and Android, is famous for his product obsession. In his first founder’s letter as CEO in 2016, he predicted that “we will move from a mobile-first world to an AI-first world.” In the years that followed, Pichai deeply embedded AI into every Google product, from Android devices to the cloud.
Despite this, the last year has largely been defined by the Artificial Intelligence launches of another company, OpenAI. The launches of DALL-E and GPT-3.5 last year, followed by GPT-4 this year, dominated the industry and started an arms race between startups and tech giants.
Gemini is now the latest effort in that race. This next-generation system was led by Google DeepMind, the newly integrated organization led by Demis Hassabis that brings together the company’s AI teams under a single umbrella. You can try Gemini on Bard today, and it will be integrated into the company’s product lineup throughout 2024.
We spoke with Sundar Pichai at Google’s offices in Mountain View, California, on the eve of Gemini’s launch, to discuss what the tool will mean for Google, its products, AI, and society at large.
The following transcript represents Pichai in his own words. The conversation has been edited for clarity and readability.
Why is Gemini exciting? Can you tell me what the big picture is that you see regarding AI, its power, its usefulness, and the direction it will take in all of your products? Sundar Pichai: A specific part of what makes Gemini exciting is the fact that it is a natively multimodal model from the start. Just like humans, it’s not just learning from text. It’s text, audio, code… So the model is innately more capable because of that, and I think it will help us discover new capabilities and contribute to the progress of the field. This is exciting. It’s also exciting because Gemini Ultra is state-of-the-art in 30 of the top 32 benchmarks, especially in the multimodal benchmarks. This MMMU benchmark shows the progress. Personally, I find it exciting that in MMLU [massive multi-task language understanding], which has been one of the main benchmarks, it surpassed the 90% threshold, which is a huge milestone. The state of the art two years ago was 30% or 40%. So just think about how much the field is progressing. Approximately 89% are human experts on these 57 subjects. This is the first model to surpass this limit.
I’m also excited that it’s finally coming to our products. It will be available to developers. It’s a platform. AI is a profound platform shift, bigger than the web or mobile. Therefore, it represents a big step for us at this time too.
Let’s start with these benchmarks. It seemed to be ahead of GPT-4 in almost all of them, or most of them, but not by much. GPT-4 seemed to be a major advance. Are we starting to plateau with what we’re going to see some of these big language model technologies be able to do or do you think we’re going to continue to have these big growth curves?
Firstly, looking to the future, we see a lot of free space. Some of the benchmarks are already high. You need to realize that, when you are trying to reach something above 85%, you are already at the limit of the curve. So it may not seem like much, but we are making progress. We will also need new benchmarks. This is one of the reasons we also looked at the MMLU multimodal benchmark. [For some of these new benchmarks, the state of the art is still far inferior. There is a lot of progress ahead. Scaling laws will still work. As we increase the size of the models, there will be more progress. When I see this in its entirety, it truly feels like we are at the beginning.
I’m interested to know what you consider to be the main advances of Gemini and how they will be applied. It is very difficult for people to imagine the leaps that will happen. We’re providing APIs, and people are going to imagine that in very profound ways.
I think multimodality will be important. As we teach these models to reason more, there will be greater and greater advances. Deeper advances are yet to come.
One way to think about this question is the Gemini Pro. It does very well in benchmarks. But when we put it on Bard, I could feel it as a user. We tested it, and favorability ratings increased significantly across all categories. That’s why we’re calling this one of our biggest updates to date. And when we do side-by-side blind evaluations, it really shows superior performance. This way, you make these better models improve the benchmarks. This makes progress. And we will continue to train and move forward from there.
But I can’t wait to get it into our products. These models are very capable. In fact, designing product experiences to take advantage of everything the models have – that’s going to be exciting in the coming months.
I imagine there was enormous pressure for Gemini to be released. I’m curious what you learned from seeing what happened with the GPT-4 launch. What did you learn? What approaches have changed during this period?
One thing, at least to me: it seems a long way from being a zero-sum game, right? Think about how profound the shift to AI is and how it’s still early days. There is a world of opportunities ahead.
But with regards to your specific question, it is a rich field in which we are all making progress. There is a scientific component, there is an academic component, which is being published a lot, seeing how models like GPT-4 work in the real world. We learn from this. Security is an important area. So in part with Gemini, there are security techniques that we learn and improve based on how the models are working in the real world. This shows the importance of several things, such as fine-tuning. One of the things we showed with Med-PaLM 2 was to take a model like PaLM, actually tune it to a specific domain, and show that it could outperform the most advanced models. And this was a way for us to learn the power of fine tuning.
A lot of this is applied when we’re working on Gemini. Part of the reason we’re taking more time with Ultra [the most advanced version of Gemini that will be available next year] is to make sure we’re rigorously testing it for safety. But we’re also fine-tuning it to really explore the features.
When some of these platforms come out and people start tinkering with them in the real world, they hallucinate or may reveal some of the private data that their models are trained with. And I wonder how much of this is inherent to the technology, given the data it’s trained on, whether this is inevitable. If it’s inevitable, what kinds of things do you try to do to limit that?
You are sure. These are all active search fields. In fact, we just published a paper that shows how these models can reveal training data through a series of requests. Hallucination is not a solved problem. I think we’re all making progress on it, and there’s more work to be done. There are some fundamental limitations that we need to overcome. One example is Gemini Ultra, we are actively red-teaming these models with external third parties who use it and who are experts in these things.
In areas such as multimodality, we want to be bold and responsible. We will be more careful with multimodal implementations because the chances of wrong use cases are higher.
But you’re right in the sense that this is still a developing technology, which is why it won’t make sense for everything. That’s why in research we are being more careful about how, when, what and where we use it and when we trigger it. They have these incredible features and they have clear shortcomings. That’s the hard work we all have ahead of us.
Do you think this will ultimately be a solved problem – hallucinations or with the revelation of other training data?
With current technology of autoregressive LLMs, hallucinations are not a solved problem. But future AI systems may not look like what we have today. This is one version of the technology. It’s like when people thought there was no way to put a computer in your pocket. 20 years ago, there were people who had very strong opinions. Likewise, looking at these systems and saying you can’t design better systems. I do not agree with this view. There is already a lot of research underway to think about how to solve these problems in another way.
You talked about how profound this change is. In some of these latest changes, such as mobile telephony, there was not necessarily an increase in productivity, which remained stable for a long time. I think there’s an argument that this may have even worsened income inequality. What kind of work is Google doing to try to ensure this change is more broadly beneficial to society?
This is a very important question. I think about this on a few levels. One thing we always focus on at Google is: how can we get access to technology as broadly as possible? So I would say that even in the case of mobile devices, the work that we do with Android – hundreds of millions of people wouldn’t have access to computing otherwise. We worked hard to come up with an affordable smartphone, maybe under $50.
So making AI useful for everyone is the framework I think about. You try to promote access to as many people as possible. I think that’s part of the issue.
We are thinking deeply about applying it to use cases that can benefit people. For example, the reason we did flood forecasting early on was because we realized that AI can detect patterns and do it well. We are using it to translate 1,000 languages. We’re literally trying to bring content now in languages that you wouldn’t otherwise have access to.
This doesn’t solve all the problems you’re talking about. But being deliberate about when and where, what kind of problems you’re going to focus on – we always focus on that. Look at areas like AlphaFold. We provide an open database for viruses around the world. But… who uses it first? Where is it sold? AI isn’t going to magically improve things on some of the toughest issues like inequality; it can exacerbate it.
But the important thing is to ensure that the technology is available to everyone. You are developing it from an early age, giving access to people and talking to them so that society can think about it and adapt to it.
Without a doubt, in this technology we participate earlier than in other technologies. You know, the recent UK AI Security Forum or the work in the US with Congress and the administration. We are trying to do more public-private partnerships, attracting academic and non-profit institutions earlier.
The impacts on areas such as jobs need to be studied in depth, but I think there will be surprises. There will be surprising positive externalities, but there will also be negative externalities. The solution to negative externalities is greater than any company. It is the role of all stakeholders in society. So I don’t have easy answers for this.
I can give you several examples of the benefits that mobility brings. I think this will also be true. We have already shown this in areas such as diabetic retinopathy. There simply aren’t enough doctors in many parts of the world to detect it.
Just as I felt that giving people access to Google Search anywhere in the world made a positive difference, I think this is the way to think about expanding access to AI.
There are things that will clearly make people more productive. Programming is a great example of this. And yet the democratization of this technology is exactly what is threatening jobs. And even if you don’t have all the answers for society – and it’s not up to one company to solve society’s problems – a company can launch a product that can drastically change the world and have this profound impact.
We never offer facial recognition APIs. But people created APIs and technology advanced. Therefore, this is not in the hands of a single company either. Technology will advance.
I think the answer is more complex than that. Societies can also be left behind. If they do not adopt these technologies, it could affect their economic competitiveness. You may lose more jobs.
I think the right answer is to deploy technology responsibly, make progress, and think about the areas where it can cause disproportionate harm and work to reduce it. There will be new types of jobs. If you look at the last 50, 60 years, there are studies by economists at MIT that show that most of the new jobs created are in new areas that have emerged since then.
New jobs will be created. There will be jobs that become better, where some of the repetitive work is freed up so that you can express yourself more creatively. You can be a doctor, a radiologist or a programmer. The amount of time you spend on routine tasks versus higher-order thinking – these can all change, making work more meaningful. And there are jobs that can be displaced. So, as a society, how can we retrain, reskill people and create opportunities?
The last year has really brought to light this philosophical divide in how people think we should approach AI. You could talk about security first or business use cases first, or “accelerationists” versus “accelerationists”. You’re in a position where you have to bridge all of these philosophies and bring them together. I’d like to know what you personally think about trying to bring these interests together at Google, which will be a leader in this field, in this new world.
I’m an optimist about technology. I have always felt, based on my personal life, a belief in people and humanity. Overall, I think humanity will leverage technology to its benefit. Therefore, I have always been an optimist. You are right: a powerful technology like AI – there is a duality to it.
This means there will be times when we move forward boldly because I think we can push the state of the art forward. For example, if AI can help us solve problems like cancer or climate change, you’ll want to do everything you can to move forward quickly. But you definitely need society to develop structures to adapt, whether it’s for deepfakes or job displacement, etc. This will be a frontier – not unlike climate change. This will be one of the biggest difficulties we will face in the next decade.
Another important and uncertain aspect is the legal landscape surrounding AI. There are questions about fair use, questions about the ability to protect the results. And it looks like this will be a big problem for intellectual property. What do you tell people who are using your products to give them a sense of security that what they’re doing isn’t going to get them sued?
Not all of these topics have easy answers. When we build products like Search, YouTube, and others in the pre-AI world, we always try to get the value exchange right. In the case of AI, it is no different. We’re definitely focused on making sure we can train on trainable data, in accordance with the law, giving people the chance to opt out of training. And there’s a layer on top of that – on what is fair use. It’s important to create value for the creators of original content. These are important areas. The internet was an example of this. Or when e-commerce started: how do you draw the line between e-commerce and normal commerce?
There will be new legal structures developed over time, I guess that’s how I would think about it as this area evolves. But in the meantime, we’ll work hard to stay on the right side of the law and ensure we also have deep relationships with many current content providers. There are some areas where this is controversial, but we are working to resolve these issues, and I am committed to working to resolve this. We have to create this win-win ecosystem for all of this to work over time.
Something that people are very worried about on the internet these days is the future of the search engine. When you have the kind of technology that just answers questions for you, based on information from across the web, there’s a fear that people won’t need to visit those sites anymore. This also appears to have implications for Google. I’d also like to know if you’re thinking about this in terms of your own business.
One of the unique value propositions we have in the search engine is that we are helping users find and learn new things, find answers, but always with the aim of sharing with them the richness and diversity that exists on the web. This will be true even during our journey with the Search Generating Experience. It is an important principle by which we are developing our product. I think people don’t always come to Seeker saying, “Answer me.” There may be one or two questions that you want this for, but you still come back, learn more, or on this journey, go deeper. We constantly want to make sure we’re getting it right. And I don’t think that will change. It is important that we have the right balance.
Likewise, if you deeply add value, there will be business value in what you are offering. We’ve had questions like this from desktop to mobile. This is nothing new to us. I feel comfortable based on everything we’re seeing and how users respond to high-quality ads. YouTube is a good example where we have developed subscription models. This also worked well.
How do you think people’s experience will change in the next year as these products start to hit the market and interact? How will their experience change?
I think a year from now, anyone who starts doing something in Google Docs is going to expect something different. And if you give them that and then put them back on the version of Google Docs that we had in, say, 2022, they’ll think it’s very outdated. It’s like, for my kids, if they don’t have spell check, they’re going to think it’s broken. And you and I may remember what it was like to use these products before spell check. But more than any other company, we have built so much AI into Search Engine that people take it for granted. This is something I learned over time. They don’t value it.
In terms of new things people can do, as we develop multimodal capabilities, people will be able to perform more complex tasks in ways they haven’t been able to before. And there will be real use cases that will be much more powerful
( source: MIT Technology Review )
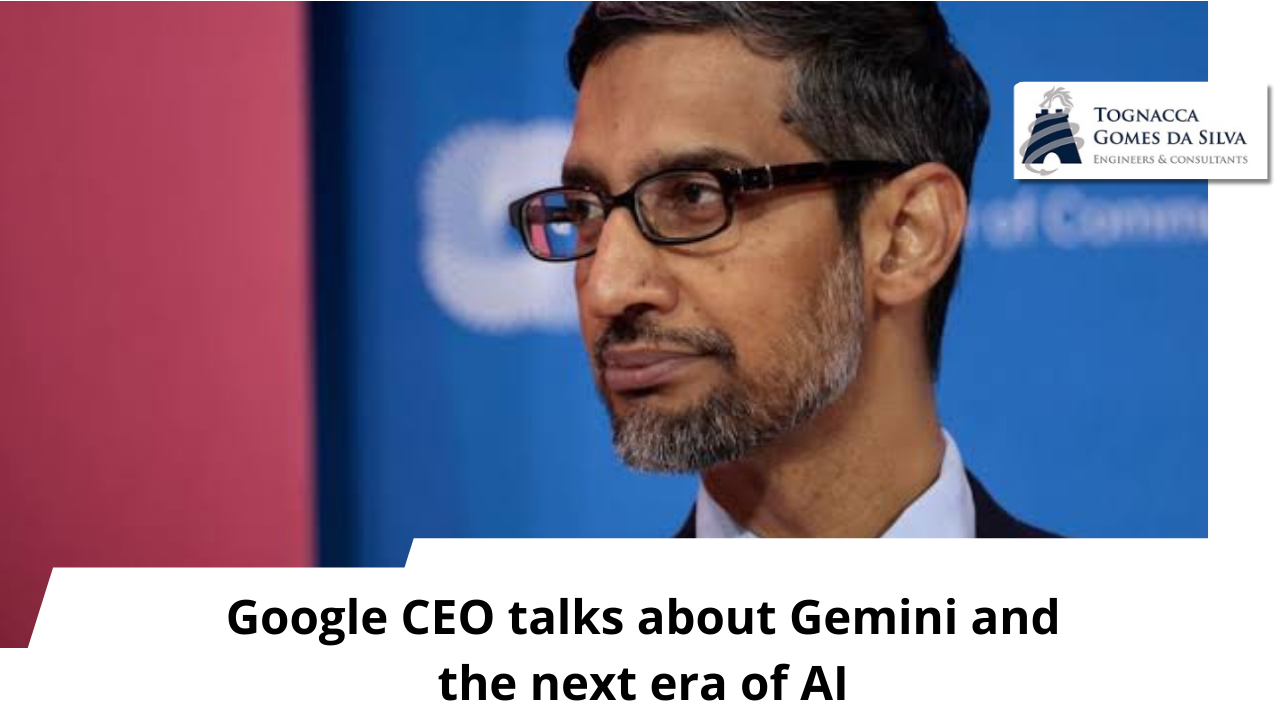