Since the general AI agent Manus was released in early March, it has spread like wildfire across the internet. And not just in China, where it was developed by Wuhan-based startup Butterfly Effect. It has entered the global conversation, with influential tech voices including Twitter co-founder Jack Dorsey and Hugging Face product lead Victor Mustar praising its performance. Some have even called it “the second DeepSeek,” comparing it to the previous AI model that stunned the industry with both its unexpected capabilities and its provenance.
Manus claims to be the world’s first general AI agent, utilizing multiple AI models (such as Anthropic’s Claude 3.5 Sonnet and tweaked versions of Alibaba’s open-source Qwen) and multiple agents operating independently to act autonomously on a wide variety of tasks. (This sets it apart from AI chatbots like DeepSeek, which are based on a single family of language models and are designed primarily for conversational interactions.)
Despite all the excitement, few people have had a chance to use it. Currently, less than 1% of users on the waitlist have received an invite code. (It’s unclear how many people are on that list, but to give you an idea of the interest, the Manus Discord channel has over 186,000 members.)
MIT Technology Review got access to Manus, and in testing it out, I found that using it was like collaborating with a highly intelligent and efficient intern: While it occasionally misunderstands what’s being asked, makes incorrect assumptions, or takes shortcuts to expedite tasks, it clearly explains its reasoning, is remarkably adaptable, and can improve substantially when given detailed instructions or feedback. In short, it’s promising, but not perfect.
Like its parent company’s previous product—an AI assistant called Monica that will launch in 2023—Manus is aimed at a global audience. English is set as the default language, and its design is clean and minimalist.
To join, users need to enter a valid invitation code. The system then directs users to a homepage that looks very similar to ChatGPT or DeepSeek, with past sessions displayed in a column on the left and a chat inbox in the center. The homepage also features sample tasks curated by the company—ranging from business strategy development to interactive learning and personalized audio meditation sessions.
Like other reasoning-based agentive AI tools like ChatGPT DeepResearch, Manus is able to break down tasks into steps and autonomously navigate the web to get the information needed to complete them. What sets it apart is a window called “Manus Computer,” which allows users to not only observe what the agent is doing, but also intervene at any time.
To test it out, I gave Manus three tasks: (1) compile a list of relevant journalists covering technology in China, (2) search for two-bedroom properties in New York, and (3) suggest potential candidates for MIT Technology Review’s Innovators Under 35 award.
Here’s how he did:
Task 1: The first list of journalists Manus provided contained just five names, with five “honorable mentions” below them. I noticed that he listed notable work by some journalists but not others. I asked Manus why. His answer was hilariously simple: He got lazy. It was “partly due to time constraints as I tried to speed up the research process,” the agent said. When I demanded consistency and depth, Manus responded with a comprehensive list of 30 journalists, listing their current outlet and mentioning relevant work. (I was happy to see my name included, along with many esteemed colleagues.)
I was impressed that I could suggest high-level changes, as if I were dealing with an actual intern or assistant, and he responded appropriately. And while he initially overlooked changes to a few journalists’ outlets, when I asked for a review of the results, he quickly corrected them. Another useful feature: the result could be downloaded in Word or Excel format, making it easy to edit or share with others.
Manus, however, struggled to access paywalled news articles; it often ran into captchas. Because I could track every step of the way, I was able to intervene to address these barriers, although many sites still blocked the tool, citing suspicious activity. I see a lot of potential for improvement here—and it would be helpful if a future version of Manus could proactively ask for help when it encountered these types of restrictions.
Task 2: For the apartment search, I gave Manus a complex set of criteria, including budget and several requirements: a spacious kitchen, outdoor space, access to Manhattan, and a major train station within a seven-minute walk. Manus initially interpreted vague requirements like “some type of outdoor space” too literally, completely ruling out properties without a private terrace or balcony access. However, after more guidance and clarification, it was able to compile a broader and more useful list, with recommendations organized into categories and tags.
The end result looked like something out of Wirecutter, with subheadings like “best overall,” “best value,” and “luxury option.” This task (including the adjustments) took less than half an hour—much less time than the journalists’ task (which took just over an hour), probably because real estate listings are more accessible and well-structured online.
Task 3: This was the most comprehensive: I asked Manus to nominate 50 people for the annual Innovators Under 35 list. Producing such a list is a huge undertaking, and we typically receive hundreds of nominations each year. I was curious to see how Manus would do. He broke the task down into steps, including analyzing previous lists to understand the selection criteria, creating a search strategy to identify candidates, compiling names, and ensuring a diverse selection of candidates from around the world.
Developing a search strategy was the most time-consuming part for Manus. Although he didn’t explicitly detail his approach, the “Manus Computer” window revealed the agent quickly browsing websites of prestigious research universities, technology award announcements, and news articles. Once again, he ran into obstacles when trying to access academic articles and content behind paywalls.
After three hours of scouring the internet—during which Manus (rightly) asked me several times if I could narrow down the search—it managed to return only three candidates with complete profiles. When I pressed it again for a full list of 50 names, it did generate one, but certain academic institutions and fields were overrepresented, reflecting an incomplete search process. After I pointed out the problem and asked it to find five candidates from China, it managed to compile a solid list of five names, although the results were skewed toward popular Chinese media figures. I eventually had to give up after the system warned that Manus’s performance would deteriorate if I continued to input too much text.
My review: Overall, I found Manus to be a highly intuitive tool, suitable for users with or without programming experience. It performed better than ChatGPT DeepResearch on two of the three tasks, although it took significantly longer to complete. Manus seems best suited for analytical tasks that require extensive research on the open web but with limited scope. In other words, it works best for the kind of things a skilled human intern might do in a workday.
Still, it’s not all smooth sailing. Manus can suffer from frequent crashes and system instability, and it can struggle to process large volumes of text. The message “Due to the high load on the service at this time, it is not possible to create tasks. Please try again in a few minutes” popped up on my screen a few times when I tried to initiate new requests, and occasionally the Manus machine would freeze on a particular page for an extended period of time.
It has a higher failure rate than ChatGPT DeepResearch — a problem the team is working to address, according to Manus’s chief scientist, Peak Ji. That said, Chinese media outlet 36Kr reports that Manus’s cost per task is around $2, which is only a tenth of DeepResearch’s. If the Manus team beefs up its server infrastructure, I can see the tool becoming a preferred choice for individual users, especially white-collar professionals, independent developers, and small teams.
Finally, I find it really valuable that Manus’s workflow feels relatively transparent and collaborative. It actively asks questions along the way and retains key instructions as “knowledge” in its memory for future use, allowing for an easily customizable agentic experience. It’s also really cool that each session can be replayed and shared.
I hope to continue using Manus for a variety of tasks, both in my personal and professional life. While I’m not sure the comparisons to DeepSeek are entirely fair, it serves as further evidence that Chinese AI companies aren’t just following in the footsteps of their Western counterparts. Rather than just innovating on top of base models, they’re actively shaping the adoption of autonomous AI agents in their own way.
( fontes: MIT Technology Review)
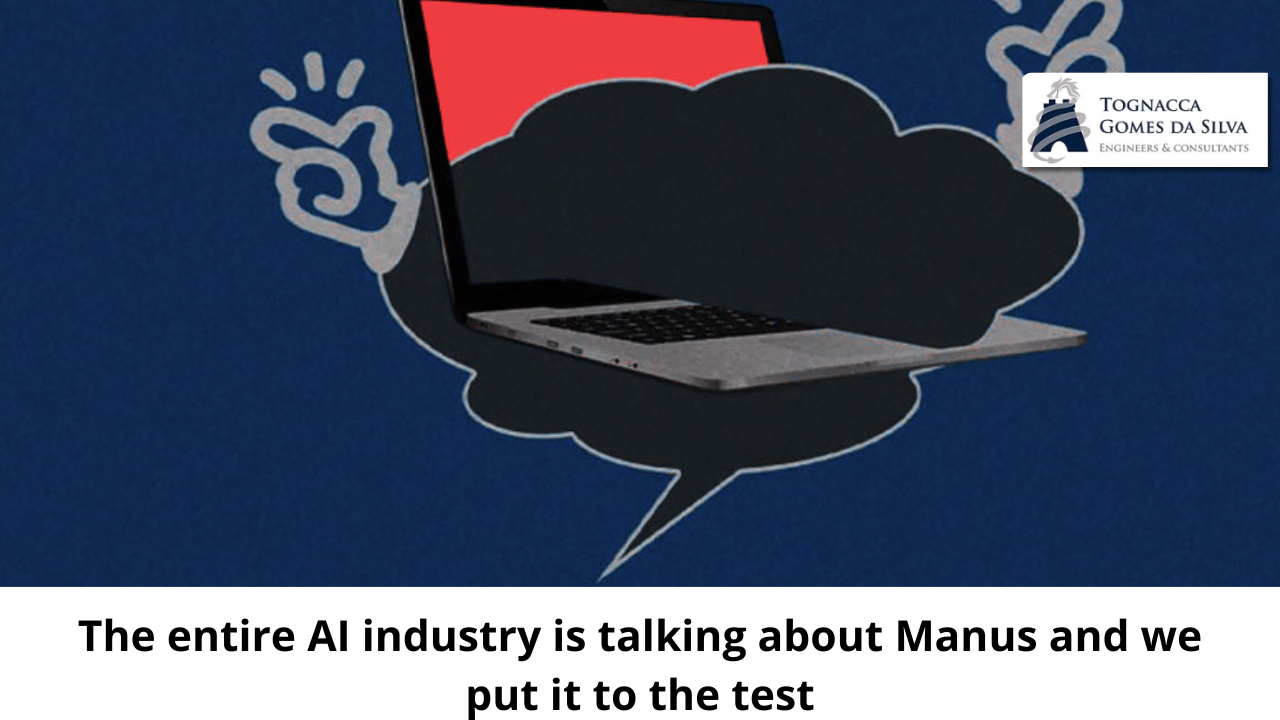