We are in the early stages of a geopolitical competition for the future of Artificial Intelligence. The winners will dominate the global economy in the 21st century. However, what is often forgotten in this conversation is that AI’s enormous demand for concentrated and consistent amounts of energy represents an opportunity to scale the next generation of clean energy technologies.
If we ignore this chance, the United States will be at a disadvantage in the race for the future of AI and energy production, ceding global economic leadership to China.
To win this race, the US will need much more electricity to power data centers. AI data centers could add the equivalent of three New York cities’ load to the grid by 2026, potentially more than doubling their share of U.S. electricity consumption to 9% by the end of the decade. Artificial Intelligence will contribute to an increase in energy demand that the US has not seen in decades. According to a recent estimate, this demand, which was previously stable, is growing at around 2.5% per year, with data centers accounting for up to 66% of this increase.
Power-hungry advanced AI chips are behind this growth. It takes three watt-hours of electricity for a ChatGPT query, compared to just 0.3 watt-hours for a simple Google search. These computational requirements make AI data centers energy dense, requiring more power per server rack and orders of magnitude more power per square meter than traditional facilities. Sam Altman, CEO of OpenAI, reportedly suggested to the White House the need for AI data centers with a capacity of five gigawatts—enough to power more than 3 million homes. And AI data centers require stable, reliable power 24/7, operating 99.999% of the time of the year.
The demands that these gigawatt-scale users are placing on the electrical grid are accelerating much faster than we can expand the physical and political structures that support the development of clean electricity. There are more than 1,500 gigawatts of capacity waiting to connect to the grid, and the time to build transmission lines to move that power now spans a decade. An example of the challenges of integrating new energy sources: the biggest factor delaying Constellation’s recently announced restart of the Three Mile Island nuclear plant is not the facility itself, but the time it takes to connect it to the grid.
The knee-jerk response to the challenge of scaling the supply of clean electricity has been to present a false choice: cede the U.S. advantage in AI or compromise our commitment to clean energy. This reasoning argues that the only way to meet the growing energy demands of the computing economy will involve expanding legacy energy resources such as natural gas and preserving coal plants.
The disastrous ecological implications of relying more on fossil fuels are clear. But the economic and security implications are equally serious.
Additional investment in fossil fuels threatens our national competitiveness as other countries advance clean technologies that represent the next generation of economic opportunities—markets measured in trillions of dollars.
The reality is that the unprecedented scale and power density required for AI requires a new set of generation solutions capable of delivering 24/7 reliable power in increasing quantities. While fossil fuel advocates have historically pointed out the variability of renewables, energy sources that require huge, distributed and uninterruptable fuel supplies, like natural gas, are also not the answer. In Texas, natural gas plants were responsible for 70% of outages following a severe winter storm in late 2022. As climate change intensifies, weather-related outages are likely to increase.
Rather than seeing a choice between AI competitiveness and the climate, we see AI’s urgent demand for energy density as an opportunity to drive a range of new technologies, taking advantage of new buyers and new market structures—positioning the U.S. not only to conquer the future of AI, but to create the markets for the energy-dense technologies that will be needed to power it.
AI’s energy density demands also require a new set of electrical infrastructure improvements—such as advanced conductors for transmission lines, which can move up to 10 times more power across much smaller areas, cooling infrastructure to handle the heat generated by chips that consume enormous amounts of energy, and next-generation transformers that enable the efficient use of high-voltage power. These technologies offer significant economic benefits to AI data centers in the form of increased power access and reduced latency, and will enable the rapid expansion of our 20th century power grid to meet 21st century needs.
Furthermore, the convergence between AI and energy technologies will enable faster development and expansion of both sectors. In the clean energy sector, AI serves as a method of invention, accelerating the pace of research and development of next-generation materials. It is also a tool for manufacturing, reducing capital intensity and increasing the pace of expansion. We are already using AI to overcome barriers in next-generation energy technologies. For example, Princeton researchers are using it to predict and avoid plasma instabilities, which have long been obstacles to sustained fusion reactions. In the context of geothermal energy and mining, AI is accelerating the discovery and development of commercial resources, as well as optimizing the performance of plants in the field.
Historically, the deployment of new clean energy technologies has depended on utilities, which are notoriously slow to adopt innovations and invest in pioneering commercial projects. Now, however, AI has brought a new source of capital to power generation technologies: big technology companies that are willing to pay a premium for 24/7 clean energy and are eager to move quickly.
These “new buyers” can build additional clean energy capacity in their own territories or can deploy innovative market structures to encourage utilities to work in new ways to scale these technologies.
We are already seeing examples, such as the agreement between Google, geothermal energy developer Fervo, and Nevada utility NV Energy to ensure clean, reliable energy at a premium price, intended for use by data centers. The emergence of these time-sensitive but price-insensitive buyers could accelerate the deployment of clean energy technologies. The geopolitical implications of this connection between AI and climate are clear: the socioeconomic fruits of innovation will flow to countries that win both the AI and climate races.
The country that can expand access to a reliable source of baseload energy will attract AI infrastructure in the long term—and benefit from the markets that AI will generate. And the country that makes these investments first will be ahead, and this advantage will expand over time, as technical progress and economic productivity reinforce each other.
Today, the clean energy scorecard favors China. The country has commissioned 37 nuclear plants in the last decade, while the US has added just two. China is investing twice as much as the US in nuclear fusion, with teams working virtually around the clock to commercialize the technology. Given that the competition for AI supremacy comes down to scalability of energy density, building a new fleet of natural gas plants while our main competitor builds an arsenal of the densest energy sources available is like bringing a knife to a gunfight.
The United States and American technology companies, who are at the forefront of the AI economy, have a responsibility and opportunity to change this by harnessing AI’s power demands to scale the next generation of clean energy technologies. The question is: will they?
Michael Kearney is a general partner at Engine Ventures, a firm that invests in startups commercializing innovative science and engineering. Lisa Hansmann is a principal at Engine Ventures and previously served as special assistant to the president in the Biden administration, working on economic policy and implementation.
( fonte:MIT Techonology Review )
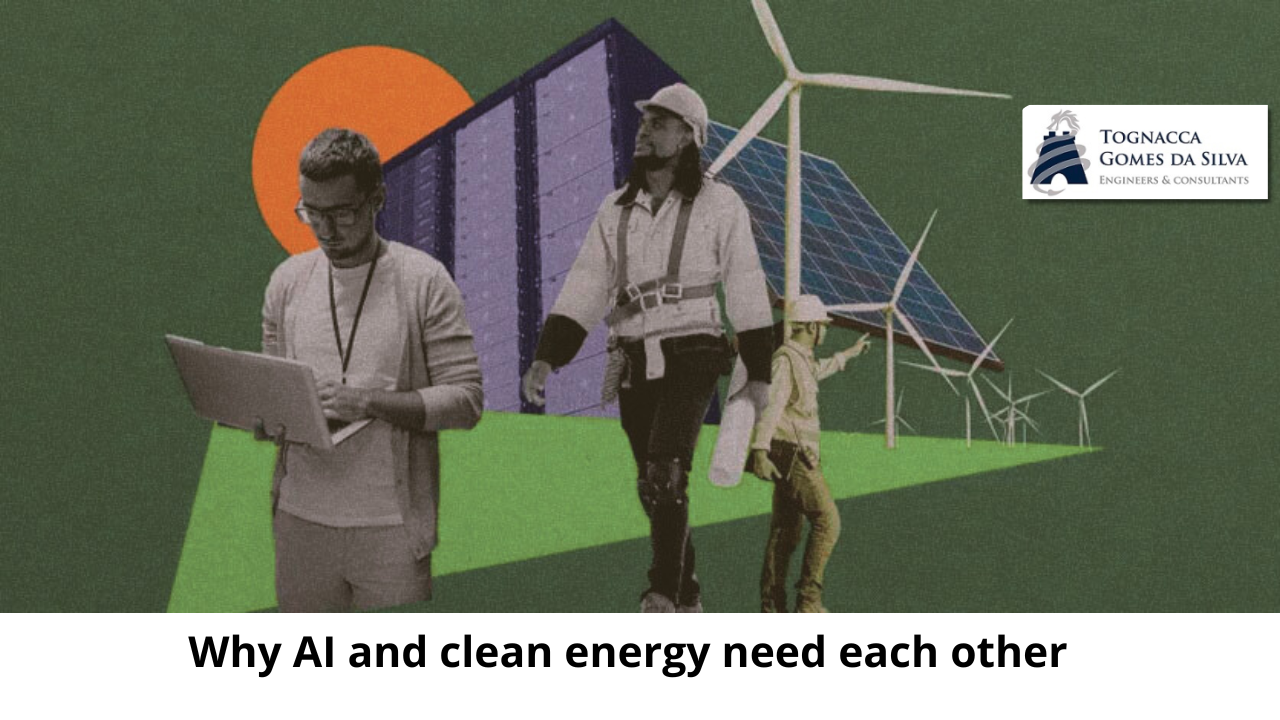